Bloomberg Ai Researchers Mitigate Risks Of Unsafe Rag Llms And Genai In Finance
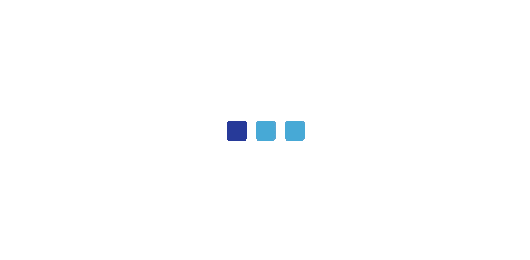
From discovering that retrieval augmented generation RAG-based large language models LLMs are less safe to introducing an AI content risk taxonomy meeting the unique needs of GenAI systems in financial services, researchers across Bloombergs AI Engineering group, Data AI group, and CTO Office aim to help organizations deploy more trustworthy solutions.
They have published two new academic papers that have significant implications for how organizations deploy GenAI systems more safely and responsibly, particularly in high-stakes domains like capital markets financial services.
In RAG LLMs are Not Safer: A Safety Analysis of Retrieval-Augmented Generation for Large Language Models , Bloomberg researchers found that RAG, a widely-used technique that integrates context from external data sources to enhance the accuracy of LLMs, can actually make models less safe and their outputs less reliable.
To determine whether RAG-based LLMs are safer than their non-RAG counterparts, the authors used more than 5,000 harmful questions to assess the safety profiles of 11 popular LLMs, including Claude-3.5-Sonnet, Llama-3- 8B , Gemma-7B, and GPT-4o. Comparing the resulting behaviors across 16 safety categories, the findings demonstrate that there were large increases in unsafe responses under the RAG setting. In particular, they discovered that even very safe models, which refused to answer nearly all harmful queries in the non-RAG setting, become more vulnerable in the RAG setting see Figure 3 from the paper.
This research clearly underscores the need for anyone using RAG LLMs to assess whether their models have any hidden layers of vulnerability and what additional safeguards they might need to add.